Why do data quality? A case study
And while the consequences of non-healthcare data quality errors aren’t usually quite as dramatic as “someone might die,” they are causing problems, right? Otherwise why are we even doing this?
- from Don’t trust the dashboard: A key question for hiring DQ team members
Why are we doing data quality?
For some of you, the answer might start with “we’re legally required to”: GDPR, among other up-and-coming data privacy regulations, requires that covered companies maintain a data quality program.
Is it worth going beyond the minimum to achieve compliance, though? And if you don’t have to have data quality, is it worth pursuing?
It is. Here’s a case study in why (plus what we did to make it happen).
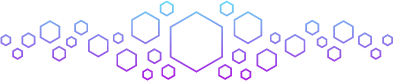
The situation
We were working with Deutsche Telekom (DT). DT is the leading German telco, with more than 151 million mobile customers, 38 million fixed-network lines, and 15 million broadband lines. Currently, they have $115 billion in annual sales.1
It’s a safe guess that a company that size probably hasn’t achieved perfect technical unity. And indeed they hadn’t.
In fact, DT had evolved a markedly decentralized approach to IT, where each department had full responsibility for its own data systems, and was wholly focused on its own niche and own customers.
As you can imagine, these departments all developed their own operational practices too.
The corporate-level documentation of all these independent operations didn’t reflect the actual day-to-day IT activities of individual departments, and not all those activities were aligned with DT’s overall understanding of its data and processes.
In 2007, DT introduced a new and ambitious master data management plan. Responsibility for data quality was given to a central data quality department, and new data governance, quality, and quality assurance protocols were introduced.
The problem
As a typical example of what the new DQ team faced, take the revenue assurance system.
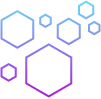
Revenue assurance systems are supposed to identify billing problems so that customers are billed exactly the right amount. Underbilling results in missing revenue, while overbilling reduces customer satisfaction and, for some enterprises, can have legal implications.
Major challenges facing data quality for the revenue assurance system included:
- Frequent changes: DT’s decentralized infrastructure let departments make architectural changes (including major ones) to their systems relatively frequently. And since departments were responsible for operations, not data quality, communication with the data quality team was sometimes lacking or slow.
- Complex systems: Department systems were frequently made up of multiple subsystems. Even within a single department, data could flow asynchronously through multiple paths, making it even harder to track down the source of an error. The revenue assurance system, for example, originated everything from a single CRM, but the data then traveled through 1-5 other subsystems.
- Missing and lost relationships: A single customer’s information was often spread across the infrastructure of several departments, making it difficult to directly compare the data. This dispersion also meant that the relationships between customers and contracts were frequently lost. The data quality program needed to reconstruct those: assessing the correctness of a customer’s bill is impossible unless you know what contracts the customer has.
- Massive data: The data quality project would need to handle 11 billion records. And, because production systems couldn’t support resource-intensive analytics alongside normal operations, the data also had to be migrated to an analysis-specific system.
Results
So let’s skip to the end: did DT’s revenue assurance actually need data quality?
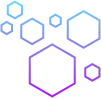
Yes. We found that 4% of billed contracts were underbilled.
The total missing revenue: $50 million/year.
There aren’t many companies where an extra $50 million annually isn’t worth it, and even if data quality requires an up-front investment, it’s usually not a $50 million investment, and costs don’t continue at up-front levels.
(If your data quality costs are that high on an ongoing basis, please get a second, third, and fourth opinion on your solution.)
In addition to the cash savings, Deutsche Telekom was also able to start catching data quality errors earlier, and customer satisfaction measurably increased. And as the cherry on top, the improved data made it possible for DT to automate many of its processes, which resulted in additional cost savings.
What it took
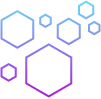
Clearly, increasing revenue, savings, and customer satisfaction took more than the minimum-effort “put up a dashboard and watch it” approach.
Instead, DT used our MIOvantage software platform to produce these results:
- A unifying central model showed the relationships between the entities that the revenue assurance system knew about.
- MIOvantage’s expansive connector tools directed each department’s data into the model. It didn’t need any preprocessing or affect the original source files, so it didn’t affect operations.
- MIOvantage’s entity resolution capabilities matched and linked data that was about the same customers, bills, contracts, and products.
- Finally, MIOvantage compared all the linked data to automatically judge which data most reliably described each entity. This “best” data was committed to the main database, creating a single set of most-reliable improved data.
Data quality that involves active intervention to improve the data is the kind that will get you the big returns.
What it didn’t take
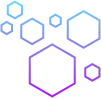
But that’s not to say that active data quality needs to actively disrupt operations--despite what all the ‘touching other systems’ described above might imply.
Operational disruption increases the opportunities for data quality failures, not to mention fomenting undesirable effects like decreased productivity and decreased customer and employee satisfaction… which is all exactly the opposite of what we’re trying to achieve in the first place.
So, MIOvantage data quality fits in around existing IT.
DT was able to take advantage of that:
- The essential central model, entity resolution operations, and data quality rules didn’t have to change whenever a new subsystem was added or removed from the data quality program. Independent connectors isolated the sources from the project’s core capabilities.
- Existing IT systems didn’t have to change, avoiding additional upset of an already very hard-to-manage technical ecosystem. MIOvantage read the data from the operational systems without affecting them.
- System users didn’t have to manually shepherd the ongoing, high-value data consolidation and relationship discovery process; MIOvantage carried it out automatically.
- DT didn’t have to commit to a long-term solution size up front or jump through hoops if they need to grow it. All scaling is handled automatically by MIOvantage’s platform architecture.
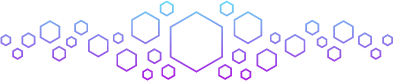
So in conclusion
Data quality beyond the dashboard can produce major positive impacts without turning your existing data ecosystem upside-down.
It's a worthwhile investment, and one that you should definitely explore if you're already having to implement some level of data quality for compliance reasons.
Obviously, not every vendor is going to be able to deliver the kind of big-upside, low-disruption results we did here.
And of the ones that can, not every vendor is well-suited to every single project. So be sure to shop around for a data quality solution that's going to be able to do these things for you.
So far, the industry has mostly focused on providing data quality for large enterprises: if that’s you, you’ll have the biggest choices of data quality provider.
If you’re coming from a small-to-medium business (or a small-to-medium department within a large enterprise), you’ll have fewer choices.
But your options are starting to increase, like with our new offering, so don’t give up if the first few data quality solutions you come across don’t look like what you need.
(PS Big or small, we’re happy to talk to you about MIOsoft data quality options no matter where you are in your data quality journey.)