Don’t trust the dashboard: A key question for hiring DQ team members
For effective data quality, you need the right mindset. If you’re interviewing data-oriented potential recruits for your project—especially data scientists and analysts—there’s one question you should always ask:
What do you think of using dashboards in data quality?
The answer should be something like: Dashboards are great for some purposes, like top-level monitoring and summarizing results for outside presentations. But they don’t provide the necessary level of detail for in-depth examinations or root cause analysis; you still need access to the underlying data.
(They probably won’t phrase it like that, but you get the idea).
If you agree, you can stop here—but if you’re not convinced, read on.
Based on their pervasive presence in popular business intelligence and analytics products, it’s clear that dashboards play a central role in data quality management.
But they’re also frequently disconnected from the actual data that the dashboard information represents. Whether that’s from technical barriers or to enable faster delivery, dashboards don’t let you look below the surface level.
For data quality, that’s a significant problem. In fact, it’s two significant problems.
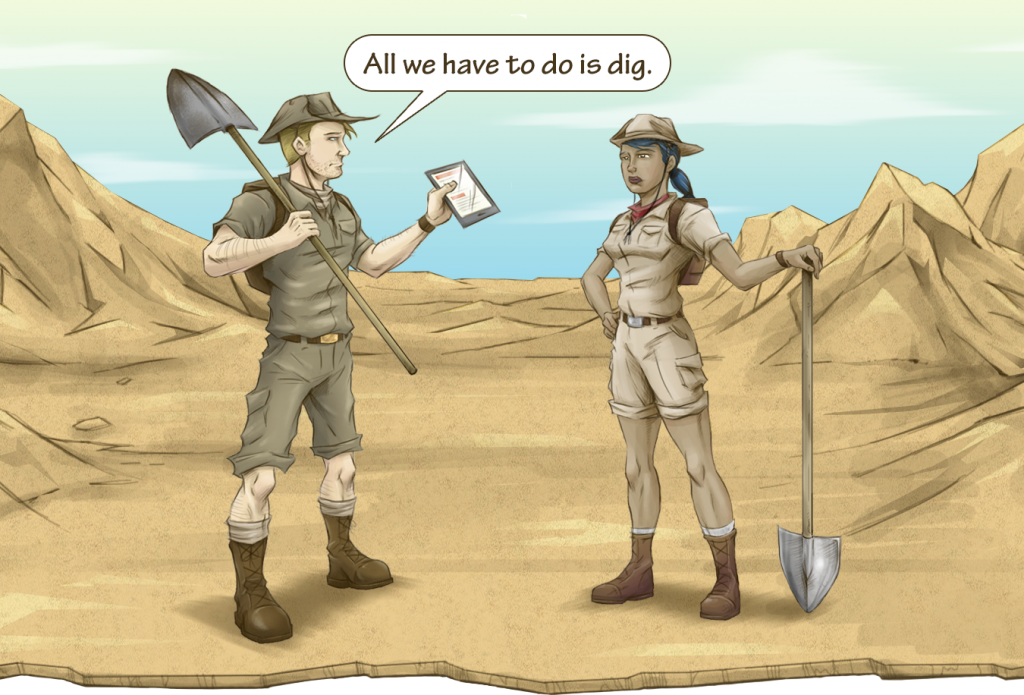
A key aspect of data quality is identifying, one, what the quality issues actually are, and two, identifying why they are. Without being able to tie specific root causes to specific types of errors, actually improving your data quality will be more a matter of chance than purposeful action.

Imagine a hospital with high failure rates for a particular set of best practices. Looking at 77% passing on a dashboard doesn’t tell you anything about what the problem is with that other 23%.
But it’s imperative to find out: peoples’ lives and health is at stake. And while the consequences of non-healthcare data quality errors aren’t usually quite as dramatic as “someone might die,” they are causing problems, right? Otherwise why are we even doing this?

So you need to dig down and see what’s going on in the actual dataset. And often, the creation of summary data dissects the dataset enough to create consumable groups of data points.
But the dashboards of popular business intelligence and analytics products, from pointed services like Google Analytics to the broad enterprise-grade offerings, don’t let you drill down that far.
So you need to reach beyond a dashboard to get that ground-level data that will let you find out exactly what is going wrong and why.

But there’s a second reason that a data quality expert should never fully trust a dashboard.
You (or someone on your team) needs to always be able to see the underlying data of a dashboard summary or calculation so that you’re always able to recalculate it.
If you’re using your dashboard to make decisions that are of any consequence at all, you must be able to verify that your dashboard is an accurate interpretation of the data. Otherwise the dashboard becomes a one-sided argument that you can never challenge, no matter how much additional context starts pointing you another way.
This is often brushed over because it requires accepting an uncomfortable truth: statistical manipulation is not that difficult. It’s all too easy for someone on the team behind your dashboard to make a mistake (in the best scenario) or actively manipulate the data (in the worst).1
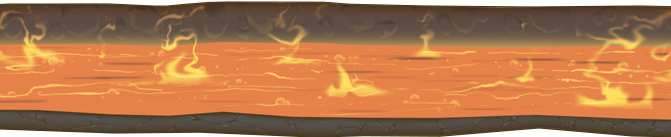
As you get more data, from more sources, going beyond the dashboard level will get more difficult.
But as your data volumes and calculation complexity rise, so do the policy and business implications of the decisions you make based on those calculations. The threat of data manipulation by a bad actor becomes more serious. Time and resources wasted as you pursue root causes down dead ends become a bigger chunk of the budget.
That’s why it’s important to make sure your data quality program doesn’t stop with a dashboard—and to make sure your team members understand why that’s important.
By hiring people who recognize the limitations of dashboards, you can build a “data first” program that has the foundational data you need for root cause analysis, error correction, forensics, validation, and deep understanding of your business.
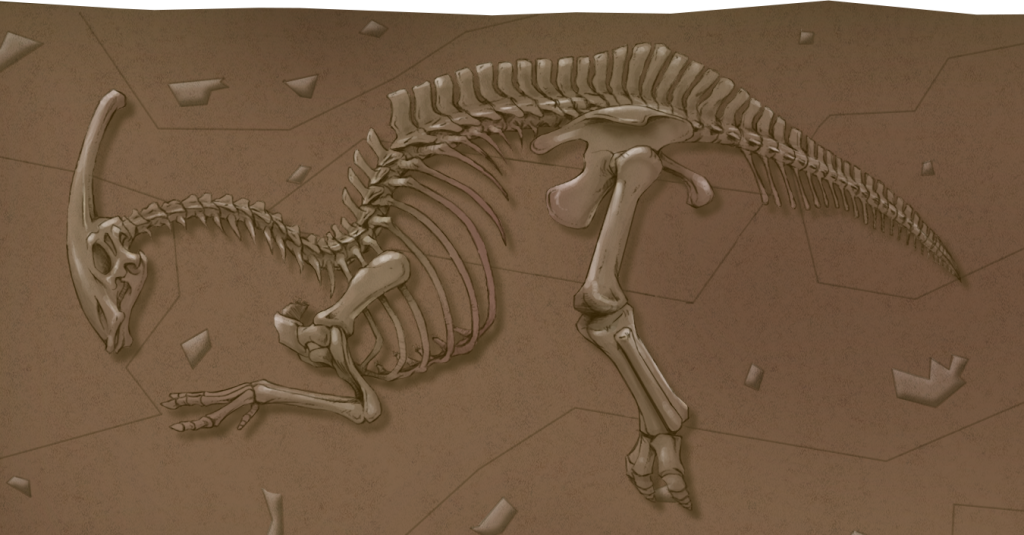
1To learn more about statistical manipulation, see Damn Lies and Statistics by Joel Best (2012) and/or How to Lie with Statistics by Darrell Huff (1993).